Abstract.
This paper studies the causal relationship between education and crime in the United States. In contrast to the majority of literature in this field, which focuses only on the effects on male crime, this study will utilise arrest rate data for both male and female perpetrators, motivated by a documented narrowing in the gender offending gap. Using changes in compulsory schooling laws as a measure of education, the study of FBI UCR data from 1974 to 2020 reveals a significant crime reducing effect of education for both genders. When using a fixed effects framework, a greater impact is found for female crime than for male. Whilst this replicates findings from studies that analyse data preceding 1980, this paper concludes that the underlying reasons are likely to be different, due to a shift in societal gender roles. The relationship is then studied further through a regression discontinuity design, examining narrower time periods around education reforms. This analysis confirms the negative causal relationship between education and crime, and offers additional insight in the form of a greater time lag for the effect on female arrests than for male. This instigates an interesting avenue for future research. Overall, the analysis of recent US education reforms supports existing evidence that education has substantial benefits beyond the market effect
Table of Contents.
1. Introduction
2. Review of Literature
2.1 Theoretical Contributions
2.2 Empirical Evidence
3. Data
3.1 Arrest Data
3.2 Compulsory Schooling Reforms
3.3 Data Caveats
4. Methodology
4.1 Addressing Endogeneity of Education
4.2 Regression Framework
5. Results and Discussion
5.1 Pooled OLS
5.2 Fixed Effects
5.3 Regression Discontinuity
6. Conclusion
7. References
8. Appendices
1. Introduction.
The existence of criminal activity in our society is extremely costly, with vast psychological, physical, and monetary impacts for the individuals affected. Although the victims may seem the most obvious of those impacted, there are also intangible consequences for offenders and the families of those incarcerated. Even those who have never directly experienced crime likely alter the way in which they live, to account for the fear of being targeted (Garofalo, 1981). In broader terms, a high prevalence of crime in an area can reduce feelings of safety, hindering the functioning of society by inhibiting community collaboration and causing residents to feel weaker attachments to their neighbourhoods (Taylor, 1995).
Given this, it is hardly surprising that crime prevention and response is a major economic activity. In 2021, the US Department of Justice received $41.1 billion of funding, with 46.6% spent on law enforcement and 26.8% on correctional facilities (US Department of Justice, 2021). These sizeable costs and potential gains from reducing the prevalence of criminal activity motivate the empirical analysis of various potential determinants of crime. These include, but are not limited to, unemployment (Raphael and Winter-Ebmer, 2001), inequality (Fajnzylber, Lederman and Loayza, 2002), legal alcohol access (Carpenter and Dobkin, 2015), police presence (di Tella and Schargrodsky, 2004), age (Buonanno and Montolio, 2009) and education.
The low average educational attainment of criminals is well-documented, with two-thirds of US prisoners having not graduated from high school (Buonanno and Leonida, 2009). Extensive research investigates whether this observation indicates correlation or causality, with the majority concluding a causal effect does exist (see, for example, Anderson, 2014 and Bennett, 2018). Despite the magnitude of papers studying the relationship between education and crime, there are gaps that can be identified in the literature. Firstly, there is a lack of up-to-date research, with few papers analysing arrest data after 2000, and the study of most education reform policies only considering those before 1980. Secondly, female crime is hugely under-represented in existing studies, which is becoming an increasingly important oversight with findings of gender-crime convergence.
In the last century, a narrowing has occurred between men and women across many fields, including labour force participation, occupations, and education (Goldin, 2014). Though perhaps occurring less rapidly, evidence shows a similar gender-convergence pattern appears to apply to criminal activity (Chen and Giles, 2004). Beatton, Kidd and Machin (2018) report this is down to faster declines in male crime rates than female, for both property and violent crimes. Currently, the majority of research focuses on men, justified by their substantially higher arrest rates (Lochner, 2020). Although this remains true, with men still committing the majority of offences, this observed convergence in criminal behaviour necessitates the consideration of both sexes when carrying out empirical research – female crime should no longer be considered trivial.
The only paper, to my knowledge, providing substantial evidence that educational attainment can affect female crime is compiled by Cano-Urbina and Lochner (2019), who study female crime up to 1980. This period was chosen in order to facilitate comparison to existing studies on males. As the role of women in society has changed drastically since 1980, partially through greater participation in the labour market and a fall in fertility rates (Hamilton and Ventura, 2006), CanoUrbina and Lochner (2019) acknowledge the possibility for a change in the effect of more recent compulsory schooling laws on female crime rates. This motivates my analysis, which will utilise established methods for testing the causal effect of education on crime, but with the inclusion of females, and for a more recent time period than is frequently considered.
The results in this paper support the existence of a crime reducing effect of education for both genders from recent reforms. This has significant policy implications, confirming that the positive crime externalities of education should be considered when evaluating the benefits of schooling. This study also offers interesting findings regarding differing impacts between men and women. Female crime appears to be affected more prominently, but there is a greater time lag before these effects are seen.
This paper proceeds as follows. Section 2 reviews relevant theoretical and empirical literature, followed by a description of the construction and limitations of data in Section 3. Section 4 outlines the model and estimation techniques, and Section 5 presents and discusses the results. Section 6 will conclude the paper, highlighting areas for future potential study.
2. Review of Literature.
2.1 Theoretical Contributions.
Since Gary Becker’s seminal paper in 1968, the study of crime in an economic framework has become an increasingly investigated topic, with the emergence of numerous studies analysing the socio-economic determinants of criminality. Becker (1968) revolutionised the perception of criminal behaviour, applying a rational choice model to a concept previously deemed to hinge on irrationality, and the inner structures of individuals. His theoretical contribution hypothesises that an individual will commit a crime if the expected utility exceeds that from allocating time and resources towards a legitimate activity. This pioneering work allowed for the subsequent prediction and analysis of how alterations in the severity and probability of punishment, and changes in the economic and social environment, can affect crime rates.
Crime and education
Becker’s model underpinned a strand of literature focussing more specifically on schooling and crime participation. Lochner (2004) designed a human capital model, attributing the effects of education on crime to labour market channels. Using the well documented theory that more schooling leads to higher potential earnings (Mincer, 1974 and Card, 1999), Lochner extends the idea of rational choice, deducing that education decreases crime rates by increasing the opportunity cost of foregone earnings from devoting time to crime instead of legal occupations. Furthermore, the cost of punishment is increased – incarceration inhibits an individual from participating in the labour market for a period of time, which is more costly for higher earners. He concludes that education must therefore reduce the incidence of property crime, but may not have such a significant effect on (or may even increase) white-collar crime1 , due to acquired skills potentially increasing the returns from such crimes more significantly than returns from the labour market. Economists have proposed alternative mechanisms to explain the crime reducing effect of education. Usher (1997) presents education to deter crime through a civic externality, detailing that school “perpetuates the values of society…and promotes the virtues of hard work and honesty”, thus having an impact beyond the pure market effect. Education may also increase patience (Becker and Mulligan, 1997). This can induce less focus on immediate needs, causing individuals to prioritise future legitimate income over present gains from criminality. These lower time preferences also imply greater weighting of the chance of future punishment, acting as a stronger deterrence to crime.
Another channel is outlined by Kling (1999), who observes that there are especially high levels of stigma following criminal conviction for individuals working in occupations with a high degree of responsibility and trust associated. Higher stigma increases incarceration costs, due to increased difficulty in returning to a previous occupation following custody time. As jobs with great responsibility tend to be higher paid, and predominantly held by more educated workers, this theory emphasises the long-term costs of imprisonment for those with greater education, which may discourage individuals from offending. Finally, education has incapacitation effects, as school attendance reduces the time available for participating in criminal activity (Witte and Tauchen, 1994). This has a clear contemporaneous effect, but can also translate to lasting effects on crime due to ‘state dependence’. As criminal behaviour has a causal effect on subsequent criminality (Nagin and Paternoster, 2006), if attending school prevents youths from committing crimes at
1The FBI defines white-collar crime as financially motivated crime, typically non-violent in nature and often committed by business and government professionals.
earlier stages in their lives, a habit of illegal activity is not built, and they may be less likely to offend once they leave education.
Assuming that education does reduce crime through one or several of the above mechanisms, there will be a social multiplier effect (Hjalmarsson, Holmlund and Lindquist, 2015). More educated individuals are likely to be surrounded by more educated peers (who are also less likely to be participating in criminal activity), hence any initial reductions in crime for the individual may be compounded by the influence of the community the person interacts with frequently.
2.2. Empirical evidence.
The first strand of empirical research addressing the relation between education and crime involved the use of standard ordinary least squares (OLS) regressions, controlling for observable characteristics. Ehrlich (1975) emphasised the importance of including parental education levels in addition to attributes of the individual, intending to control for genetic and social background factors. His cross-sectional study used data from the 1960 US Census, and modelled education by the mean number of school years completed by the population of 25 years of age and over within each state. The empirical analysis obtained mixed, and sometimes unexpected results, including a positive relationship between education and property crime. Ehrlich (1975) suggests this may be due to a negative correlation between education and unreported crime – if the general public possess higher average education levels, inefficiency of crime reporting may be decreased. However, interpreting the results of this paper (and other similar studies using cross-sectional OLS regressions) in a causal sense must be treated with caution (Lochner, 2020). There is potential for reverse causality or omitted variable bias through unobservable characteristics, as education cannot be considered exogenous in relation to crime rates.
Addressing Endogeneity of Education
To account for the endogeneity of education, much of the literature exploits exogenous changes in compulsory schooling laws2 . These contributions attempt to uncover a causal effect of education on crime rates and eliminate the possibility of spurious correlation. In their seminal paper, Lochner and Moretti (2004) adopt instrumental variable (IV) estimation, using changes in state compulsory schooling laws, to investigate this effect for men in the US. They form a panel comprising data from 1960-1980. Multiple variables are adopted to model crime – arrest data from FBI Uniform Crime Reports, incarceration data from the US Census, and self-reported crime participation measured in the National Longitudinal Survey of Youth. Regressions using these three crime indicators all produce similar conclusions, illustrating a significant crime reducing effect of schooling. Two-stage least squares (2SLS) estimates find a one-year
increase in average education to reduce state-level male arrest rates by 11%, and reduce the probability of a jail sentence by 0.1 percentage points for white males and 0.4 for black males. They do not empirically analyse the different channels through which this may have occurred, but reference other studies and conclude that a significant portion of this effect is likely through human capital improvements, and the increased wages and labour market opportunities associated with schooling. Lochner and Moretti (2004) also use their results to quantify the social returns to education with respect to crime reduction, estimating the positive externalities at $1,170-$2,100 per additional male graduate.
Empirical research using schooling reforms also spans other countries, highlighting the reproducibility of results across nations. Machin, Marie and Vuljíc (2011) use the 1972 increase in the UK compulsory schooling age from 15-16 to estimate educational effects on property and
Here, compulsory schooling laws refer to reforms increasing the mandatory minimum school leaving age.
violent crimes in England and Wales. They construct an arrest rate variable using the Offenders Index Database and Office of National Statistics population data, and carry out research using both IV and regression discontinuity methods. This study uncovers a robust negative effect of education on property crime, but results for violent crime show no clear pattern. When analysing by gender, they find a significant crime reduction from male education, but imprecise results for women, attributed to the low female arrest rates in certain age cohorts. These results are consistent with findings of Hjalmarsson, Holmlund and Lindquist (2015) for the 1950 Swedish Education Reform, and Groot and van den Brink (2010) for Denmark. The education policy in Sweden is also found to have significant effect for the children of those affected, identifying an intergenerational effect of education on crime rates (Meghir, Palme and Schnabel, 2012).
Although the majority of the causal literature exploits changes in compulsory attendance, other instruments have been utilised to study the effects of education on crime rates where schooling laws either do not offer sufficient variation, or do not help to isolate the precise channel being investigated. For example, Jacob and Lefgren (2003) investigate the incapacitation effect of education, so capture its short-term impact by studying the consequences of school days missed (induced by teacher strikes or in-service days). They estimate that lengthening the school year by one day decreases property crimes on that day by about 14%, but increases violent crime due to increased juvenile interaction. Asante and Bartha (2022) study Sub-Saharan Africa (SSA), identifying that the greatest barrier to accessing basic education in these developing countries are financial constraints. Hence, rather than using attendance laws, they use cost elimination (policies designed to offer free education to youths) as an instrument for enrolment. They find a robust negative relationship between enrolment and property crime, but again no significant result for violent crimes (as in Machin, Marie and Vuljíc (2011)).
Recent US reforms.
More recent reforms differ from those in the past by raising the mandatory dropout age from higher initial levels. Hence, there is value in analysing these, to test whether education still induces crime reductions at higher ages. The only paper analysing the impact of more recent US schooling laws is carried out by Bell, Costa and Machin (2022), who exploit changes induced by post-1980 reforms to test the effects of recent education on male crime rates in the US. An earlier version (Bell, Costa and Machin, 2016) concluded that the traditional use of 2SLS was weaker for more recent reforms, hence the updated paper uses a reduced form model with fixed effects, followed by regression discontinuity techniques.
The paper models crime using FBI UCR Data (as in Lochner and Moretti, 2004), to construct a panel from 1975 to 2015, aggregating crimes into violent, property and drug categories. The reforms are found to reduce young male arrest rates by 10% in the fixed effects model, and 6.1% when examining a more precise discontinuity window around the reforms. This study then investigates specific channels, separating the effects on crime for those directly constrained by reforms (incapacitated by attending school) and those who are no longer directly constrained. Significant negative estimates are found for both groups, suggesting the existence of both an incapacitation effect, and longer-term crime reducing effects.
I shall reproduce parts of the initial methodology used in this paper, extending the analysis to include the female population. This enables the study of any emerging differences between gender effects, in addition to decompositions by crime type and nature of reforms. I will also analyse arrest data up to 2020. The time extension will provide a longer period for analysis after the more recent reforms. For example, the most recent reform only occurred in 2010, so the Bell, Costa and Machin (2022) paper could only access 5 years of subsequent data.
Table B1 in the Appendix summarises the features of core empirics in this review.
3. Data.
This section provides a brief description of the data used in this study, indicating sources of dependent and independent variables, and potential limitations. Further details regarding variable construction and covariate information can be found in Appendix A.
3.1 Arrest Data.
This study models crime using arrest data from the FBI Uniform Crime Report (UCR). The UCR provides monthly arrest numbers from 1974 to 2020 at a local police agency level, grouped by age and sex of the perpetrator3 , and the offence committed. As this study requires the identification of whether offenders were subject to compulsory schooling reforms, offenders’ ages at the time of the crime must be known. The UCR only reports arrests by single year of age for those aged between 15 and 24, hence the analysis has been conducted on these subjects only. As the majority of crime is committed between these ages, with crime-age profiles deemed to peak at ages 19 to 20 (Bindler and Hjalmarsson, 2017), this should not interfere greatly with analysis.
UCR arrest figures cover only index crimes – eight crimes chosen for publishing by the FBI due to their strength of reporting or ability to represent overall crime incidence. Following the majority of crime literature, I categorise these crimes by property or violent nature4 , using official UCR offence identification. A list of the index crimes and their corresponding categories is given in Table 1.
The UCR does not report the race of perpetrators, hence does not allow for the examination of the effects of education on crime
along a racial dimension. 4 In crime literature, drug-related crimes are often used as a third category. However, due to the limited accessibility of data, these
are omitted from my analysis.
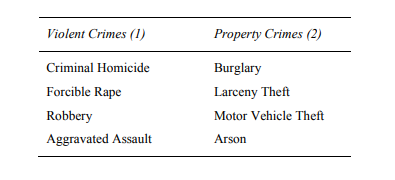
I have constructed a county-level panel by aggregating arrest figures for each police agency within each county for the 46 years in the sample. It is key to note that some agencies do not report, and others fail to report consistently or for a substantial number of years. To address this, I follow the approach commonly adopted in previous literature, removing any observations for years where an agency fails to report 12 months of data, and only including agencies that report at least 10 years in the sample. Detailed coverage information is reported for each included state in Table B2 of the Appendix.
In order to formulate arrest rates from the absolute values provided in the UCR, population data with the same demographic decomposition is required. This is available for our sample years in the Surveillence, Epidemiology, and End Results (SEER) Program of the National Cancer Institute (NCI), which presents estimated yearly data on county populations by age and sex. I then adjusted these age-sex-county population statistics according to the reporting coverage for the county5 . Arrest rates are calculated by dividing the number of arrests by the adjusted population. Calculated arrest rates of 40% and above6 were removed to avoid distortion from outliers. The logarithm of the total arrest rate is used in this study, to allow the interpretation of estimates as a percentage change.
Appendix A covers the details of this process.
Bell, Costa and Machin (2022) find this is robust to alternative thresholds of 30% and 50%.
The overall number of observations is 4,336,301, covering 46 states and 2,351 counties for the 46 years7 . Each observation corresponds to a group of offenders of a particular age, sex and crime type committed, in a given county and year.
3.2 Compulsory Schooling Reforms
Compulsory schooling law data was taken from Bell, Costa and Machin (2022), who collected information from statutes in the Westlaw International Database. When accessible, they use the stated effective date of the new law as the date of reform. However, in the cases where this is not provided, they approximate with the year of enactment.
More recent reforms often include education grades which exempt children from staying in school until the stated age. Hence, Bell, Costa and Machin (2022) consider these when formulating the new dropout ages, using the equation:
where DA gives the dropout age used in the analysis for birth cohort (t-a )in state S , where t is
year and a is age.
Bell, Costa and Machin (2022) raise concerns regarding states with multiple education reforms in a small-time proximity, and the potential for resulting spill-over effects. To address this, I have narrowed the sample to only include states that experience one schooling reform within the time frame. Figure 1 illustrates these changes in dropout ages across states, and a comprehensive list of the 19 reforms analysed and their corresponding calculated dropout age are outlined in Table B3 of the Appendix.
This sample size will vary, however, depending on the model of choice and how many years are included in the sample
All the variables used for analysis are outlined and described in Table 2 below.
Table 2: Description of variables
Variable | Purpose in Regression | Description |
---|---|---|
Log Total Arrest Rate | Dependent variable | Natural logarithm of total arrests divided by the adjusted age-sex population |
Schooling laws | Explanatory variable, instruments for education | Dummy variable indicating whether offenders’ birth cohorts were subject to a compulsory schooling reform |
Crime Type | Identifier variable | Dummy variable for type of crime committed, grouped into property and violent categories |
Sex of Perpetrator | Identifier variable | Dummy variable for sex of offender |
Year | Fixed effects | Year in which arrest was made, 1974 to 2020 |
Age of Perpetrator | Fixed effects | Age of perpetrator, ranges from 15 to 24 |
Fips State Code | Fixed effects | US Federal Information Processing Standard code – uniquely identifies each state |
Fips County Code | Identifier variable | US Federal Information Processing Standard code – uniquely identifies each county |
Time | Running variable for discontinuity estimates | Indicates number of years the birth cohort was pre/post reform, allows for the study of different bandwidths |
Adjusted age-sex-county population | Used as a weight and to calculate arrest rates. County population by age and sex, adjusted according to coverage variable | |
Share of Females | Control Variable | Share of the age-county population that is female, using SEER data |
Share of Black | Control Variable | Share of the age-sex-county population that is black, using SEER data |
Share of Other | Control Variable | Share of the age-sex-county population that is neither white nor black, using SEER data |
Log Police | Control Variable | Natural logarithm of the total number of sworn police officers from FBI LEOKA |
Log Population | Control Variable | Natural logarithm of the official county population count, from SEER data |
School Attendance | Used to confirm instrument relevance. Proportion of US Current Population Survey subjects enrolled in full-time education |
Notes: Additional information on control variables, such as sources and construction detail, is included in Appendix A.
Summary statistics for the main continuous variables are displayed in Table 3, and dummy and
categorical variables in Table 4.
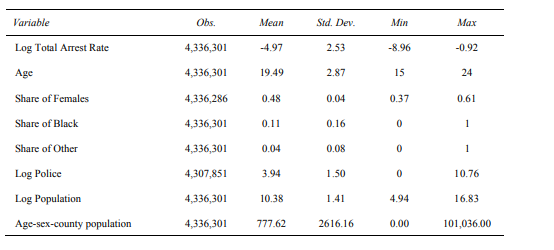
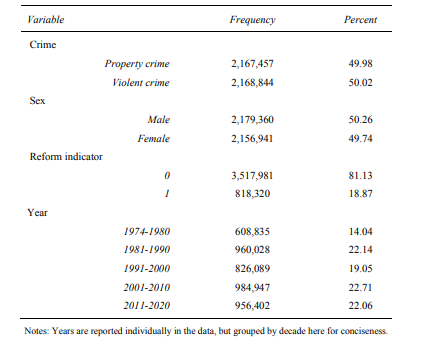
The dependent variable used in this study is the log of the total arrest rate. This has a mean of -4.97 over the sample period, which translates to an average arrest rate of 0.007. The explanatory variable is a dummy indicating whether the offender’s birth cohort was subject to a compulsory schooling reform. 18.87% of observations represent offenders who were subject to such a reform. The mean share of females in a county is 48%, with the average share of the population being black and nonwhite/non-black being 11% and 4% respectively.
The data can first be used to confirm the occurrence of a gender-crime convergence. This is a key motive for this study, hence it is vital that the data used supports this pattern. Table 5 shows the trend in the percentage of arrests with a female offender over the sample period. Between 1975 and 2020, this increases from 15.78% to 31.07%. This clearly supports claims of gender convergence, validating the importance of the inclusion of females in the analysis.
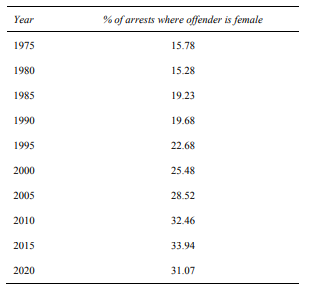
Figure 2 further illustrates the narrowing of the gender-crime gap graphically, depicting the rapidity of the decrease in total arrest rates in comparison to the female arrest rate.
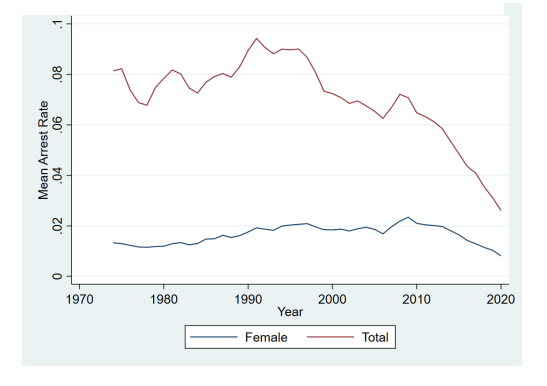
3.3 Data caveats.
Before outlining the research design, and conducting the associated empirical analysis, it is important to consider the potential caveats of this data.
Firstly, the use of arrest data to model crime has its limitations. Not all offences result in arrests, and hence arrests will never be a perfect representation of crime incidence. For certain crimes, there may be a significant degree of underreporting – Tjaden and Thoennes (2006) estimate that less than 20% of adult rapes are reported to the police. However, arrests do show strong correlation to crime and are therefore utilised in the majority of crime study. Lochner and Moretti (2004) model this association formally, assuming that arrests are a direct function of the amount of crime c committed at time t.
This model includes schooling s as a factor that could influence arrests, following concerns raised by Ehrlich (1975) regarding increased educational attainment improving the chances of individuals evading arrest after committing a crime, causing a sample bias. However, Lochner and Moretti (2004) disprove this as a key issue, using self-reported survey data. They still find similar crime reducing effects, verifying that the outcome cannot wholly be attributed to increased success in avoiding arrest.
More specifically, UCR data has several issues. Firstly, the voluntary nature of the data submission means that not all agencies report in every time period. This non-participation may not be entirely random, and hence this could affect the validity of results. In addition, crimes are grouped by the police agency governing the area in which the crime was committed, not where the offender resided when completing high school. Hence, there will be observations where the arrestee is incorrectly included in analysis for a certain reform, despite not being affected by it. This will limit the precision of the compulsory schooling instruments.
However, the fact that prominent studies continue to use arrest rates from the UCR as a measure of crime indicates the caveats of the data can be viewed as limited, and should not greatly hinder the validity of results.
4. Methodology
3.1 Addressing endogeneity of education.
Aiming to uncover the causal effect of education on crime using basic OLS regression with years of schooling as the explanatory variable suffers from clear endogeneity problems. Unobservable characteristics such as individual discount rates or degree of risk aversion are likely correlated with both schooling decisions and propensity to commit crime. Hence, a negative relationship between the two variables would be estimated, even if no causal effect is present. Reverse causality presents another issue, as juvenile incarceration from criminal activity inevitably reduces time spent in education (Hjalmarsson, 2008). This causes empirical difficulty – a negative correlation would be seen, but it is difficult to eliminate the portion driven by criminality influencing education.
I will address endogeneity issues by exploiting a natural experiment of variation in state compulsory schooling laws. This instrument is frequently used to examine the effect of education on a variety of economic outcomes, such as health (Kemptner et al., 2011), earnings distribution (Brunello et al., 2009), fertility (Cygan-Rehm and Maeder, 2013) , happiness (Dursun and Cesur, 2016) and religion (Hungerman, 2014). Schooling reforms are also a prevalent explanatory variable in education-crime studies, as outlined in the literature review. Despite the validity of this variable seeming likely on account of its use in similar papers, I have additionally confirmed the necessary requirements using my data.
Relevance
For an instrument to be relevant, it must be significantly correlated with the endogenous regressor. In this case, changes in compulsory schooling laws must provide sufficient information explaining the variance in education. Table 6 presents estimates confirming this, showing significant positive effects of the pooled reforms on high school attendance for all discontinuity windows. When using the smallest window of 5 years either side of the law introductions, reforms increase high school attendance by 1.3 percentage points.
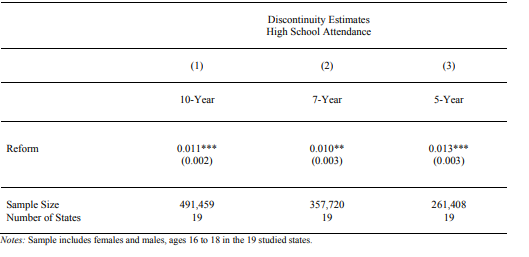
Exogeneity
Secondly, the instrument must be exogenous, meaning it is uncorrelated with any unobservables that also affect the outcome. Conditional on education levels, compulsory schooling law changes should have no independent association with crime rates. I first validate this using evidence from other papers, followed by tests with my own data.
Oreopoulos (2007) reports there is no suggestion that the enaction of education reforms were in
response to crime concerns – crime outcomes were an unintended beneficial by-product, rather than
a consideration in their implementation. This allays the concern that the timing and location of
reforms simply reflect underlying crime patterns. Another potential issue could be a correlation
between the enactment of laws and prior schooling trends. Lochner and Moretti (2004) examine
the existence of a selection bias, hypothesising that states experiencing faster increases in high
school graduation rates may incur lower costs of adopting new compulsory schooling laws, and hence are more likely to introduce them. By examining the relationship between future compulsory
attendance laws and current graduation rates, they confirm this is not the case, and reforms are not
correlated with previous academia.
To further substantiate the validity empirically, I conduct a balancing test comparing the observable covariates before and after reforms. The results in Table 7 show very little significant evidence to suggest the reforms correspond with other socio-economic changes influencing crime, with the only significant difference being the share of the population that is neither white nor black. However, it is clear that these observables do not cover all potential variables, and there could potentially be patterns in un-observables – this evidence is supportive rather than conclusive.
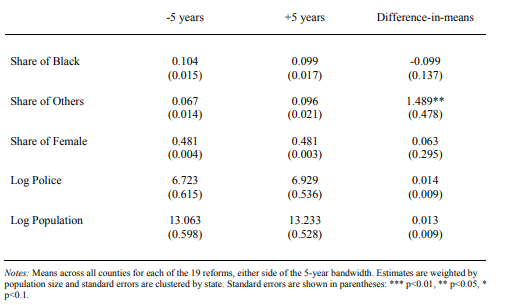
It is important to note that the use of this instrument means that our estimates represent a local treatment effect, only applying to those whose schooling decisions are altered by the law changes. Those who would have continued to pursue education past the mandatory leaving age are not represented.
4.2 Regression Framework
Pooled OLS
I begin by analysing the relationship between education reforms and crime by pooling the data and using basic OLS estimation. The following equation can be used to estimate the impact of reforms on arrest rates for individuals of age a, located in county c and state s, in time period t:
Clustered standard errors by state are included to allow for serial correlation and changing variances within each cluster. Abadie et al. (2017) define the motivation for clustering adjustments as the existence of correlated unobserved components in outcomes for units within clusters. This is evidently the case in this study, where there will be unexplained variation in crime outcomes which are correlated for counties within the same state
Covariates consist of the share of the population that is female, black or other; the log of police officers, and the log of the county population.
across county/states and time. In this study, this assumption will not hold – there will undoubtably be heterogeneity through unobserved factors that vary across counties or states and affect crime outcomes. Education reforms and the observed covariates will by no means be the only determinants of arrest rates that vary with location – there could be policing reforms, a difference in state resources, alterations to criminal justice systems etc. There will also be unobserved variables that evolve over time, such as changes to the economic cycle. Hence, the incorporation of fixed effects is necessary to remove this omitted variable bias.
Fixed Effects
Equation (2) illustrates the fixed effects regression model to be used in the analysis.
Regression Discontinuity
Although the incorporation of fixed effects is the most prominent model in the literature, I also conduct a regression discontinuity design, as in Bell, Costa and Machin (2022). This is allowed for by the sharp discontinuity in mandatory schooling generated by the education reforms. A narrower focus on years surrounding the reforms helps to limit the influence of changes in other variables on the regression. There is a low concern regarding selection bias, as short of migrating to states where laws are not being enacted, it is not possible for individuals to manipulate their selection into treatment – birth cohort is a pre-determined variable. This method is also facilitated by the large sample size, enabling the focus on observations close to the discontinuity point without reducing the power of the study to insufficient levels.
This design can be represented by the equation:
For both fixed effects and regression discontinuity models, estimates will first be processed
analysing the whole population, followed by the male and female populations separately. Maleonly effects can be used for direct comparison with results in Bell, Costa and Machin (2022), whilst
coefficients for female arrests represent a new contribution, analysing a period that has not yet been
studied for female crime.
5. Regression Results and Discussion.
5.1 Baseline model.
Table 8 presents pooled OLS estimates of the baseline model, without the inclusion of fixed effects – the specification given in equation (1). All schooling reforms are represented by one indicator variable – the reform coefficient represents the weighted-average effect of all the laws outlined in Table B2.
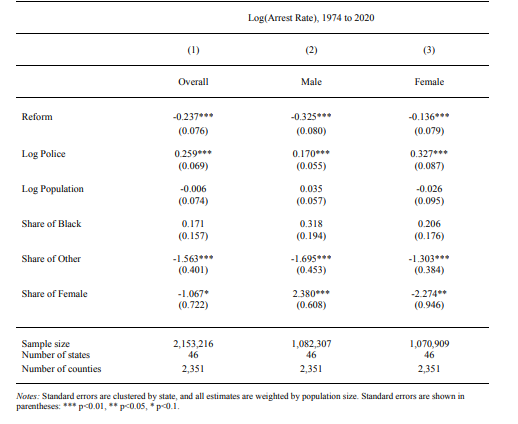
On average, the schooling reforms are associated with a 32.5% decrease in male arrest rates and a 13.6% decrease in female rates. Both these estimates are significant at the 1% level. However, these findings should not be considered to indicate a larger negative causal effect for men than for women. The issues with this model outlined in Section 4 mean that although the regression is useful as a baseline to identify the overall direction of the relationship, it should not be interpreted causally.
5.2 Fixed effects models
Problems with the utilisation of pooled OLS necessitate the modification of the regression framework to control for time, county, and age fixed effects. Equation (2) resembles the typical estimator utilised in seminal economic crime literature, and hence this model can be used to test whether the findings from existing studies are extendable to samples including females and for more recent reforms. Estimates from this model are presented in Table 9.
The first column of Table 9 indicates a reduction in overall arrest rates by 14.5% as a result of the introduction of compulsory schooling laws. The reform coefficient is significant at the 1% level. This shows that incorporating females within the arrest rate sample data still provides estimates indicating a strong crime reducing effect of education, replicating the common finding in the literature. This is unsurprising, with no theoretical contributions suggesting education should increase female crime.
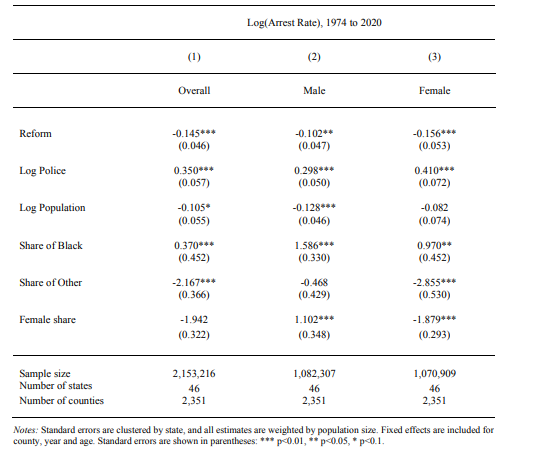
Table 9 then presents the results for each gender in columns 2 and 3. The estimates suggest the education reforms significantly reduced male arrests by 10.2%, an almost identical estimate to the 10.7% reduction found by Bell, Costa and Machin (2022). This demonstrates success in reproducing their dataset and model, and indicates that limiting the number of reforms analysed and extending the sample period evokes little change to fixed effects results.
The key contribution from this paper, isolating the effects of recent education reforms on female crime, is shown in the third column. Here, an estimated 15.6% decrease in female arrests is induced by compulsory schooling laws. The larger crime reducing effect of education on females compared to males mirrors results found by Cano-Urbina and Lochner (2019), who study pre-1980 data. This indicates that the finding that education affects female crime more substantially likely still applies in more recent years.
Pre-1980, the larger effect for females is attributed largely to increased marital prospects (CanoUrbina and Lochner, 2019). Increased schooling led to women being more likely to marry, and have more children, which discourages crime by increasing the personal cost of incarceration through strengthened familial bonds. Today, however, we see the reverse – more educated women have later first births and a higher probability of remaining childless (Kravdal and Rindfuss, 2008). Thus, the marital prospects explanation seems far less applicable. With the position of females in society constantly evolving and becoming in many regards more similar to men, it seems there is a significant possibility that the way in which female education translates to lower crime rates has changed.
Cano-Urbina and Lochner (2019) find little effect of schooling on female labour supply for their time period. However, there is now a significant positive relationship between female education and labour market outcomes. Ionescu (2012) even finds greater labour market returns from an additional year of schooling for females than for males. This suggests the greater crime reducing effect of education for women from recent reforms likely now stems from increased impacts through labour market channels. Overall, although the general pattern remains the same as in more dated research, this by no means implies similar underlying channels.
Crime Type
Table 10 presents estimates further decomposing the data by crime type, to uncover any differential effects between the two categories. This analysis is commonly carried out throughout the literature.
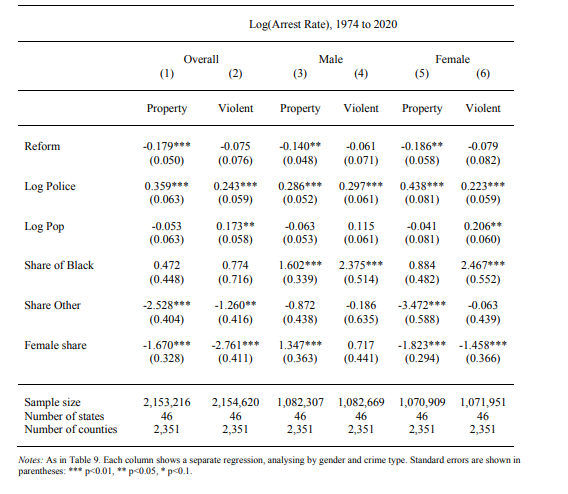
The results reveal that schooling reforms led to significant reductions in both female and male arrest rates for property crimes. Again, the estimate for female crime exceeds that of males – a 14% reduction is found for male property crime compared to an 18.6% reduction for females. Violent crime is much less precisely determined, with the analysis offering no significant evidence that education affects rates of violent crime for either gender. This is consistent with results in Machin, Marie and Vujic (2011) for the UK and Asante and Bartha (2022) in SSA. The coefficients for violent crime are also much smaller than for property. This could substantiate findings from Jacob and Lefgren (2003) that increased juvenile interaction associated with school attendance leads to more conflict and violence. The typical crime reducing effect of education may be negated due to
the social nature of violent crime.
Placebo Test
To verify the validity of the fixed effects model, a Placebo test can be implemented. This falsification method is designed to check for an association that would be present if the design was flawed, but not otherwise (Eggers, Tunon and Dafoe, 2021). By lagging the reform variable, I have generated false reforms. Regression results using these reforms can be seen in Table 11.
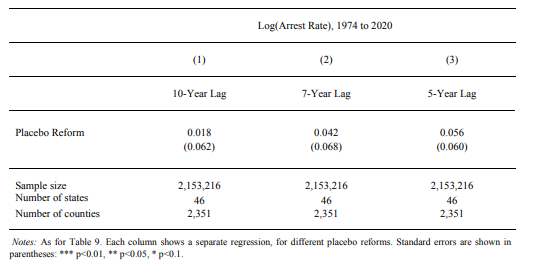
None of the coefficients relating these placebo reforms to the arrest rate are significant, which supports the suitability of the model, disproving the likelihood that the relationship found is due to other differences between states experiencing reform and those that do not, rather than educational effects.
Overall, this method appears to generate significant and reliable estimates, showing similar results to studies on earlier periods, with larger effects for females than males, and a lack of evidence for violent crimes
5.3 Regression Discontinuity Design.
To further extend the analysis and follow additional techniques adopted in literature examples, I implement a regression discontinuity design, exploiting the precise cut-off generated by each reform. Table 12 shows regression discontinuity estimates, using equation (3) to examine precise windows around the education reforms
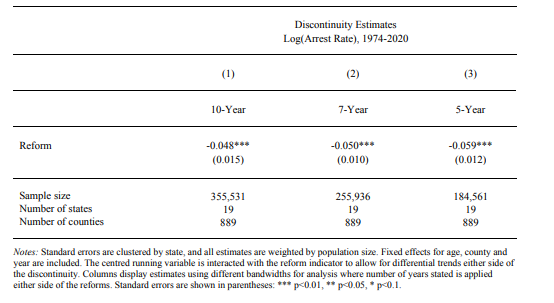
As in Bell, Costa and Machin (2022), discontinuity estimates fall substantially compared to the fixed effects estimation, but still display a significantly negative causal relationship between schooling reforms and arrest rates. Robustness is probed here by using a variety of windows around the discontinuity. As all results are significant and within a relatively small range, I proceed to adopt the 5-year window, as a narrower bandwidth focuses more tightly on the discontinuity and minimises the potential for the influence of other factors. This estimate shows compulsory schooling reforms induced a 5.9% reduction in overall arrest rates. I also experimented with different functional forms of the running variable (time), concluding that all were significant, indicating robustness to different specifications. I adopt the linear form, as recommended in Gelman and Imbens (2019). Details of these estimates can be viewed in the Appendix, within Table B4.
Reform Type
The 19 reforms can be categorised by initial minimum dropout age and dropout age after the reform is enacted. Table 13 shows the discontinuity estimates split for reforms increasing the minimum dropout age from 16 to 17, 17 to 18, 16 to 18 and all other reforms.
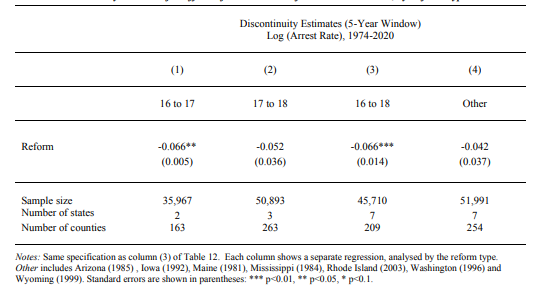
This shows reforms that increase the dropout age from 16 to 17, or 16 to 18 generate a highly significant negative effect on arrest rates, but raising it from 17 to 18 does not. This could indicate greater effect for reforms that increase school leaving age from lower initial levels, supported by the fact that Lochner and Moretti (2004), who study earlier compulsory schooling laws with far lower initial ages, find larger reductions than these.
The compilation of panel data at a county level rather than state also allows the study of each reform individually. Of the 19 reforms analysed, 13 show a negative effect and 8 are significant. This indicates that although when aggregated, compulsory schooling reforms appear to have great effect, not every reform may have had significant impacts. These results are presented in Figure 3, whilst the precise coefficients and standard errors are reported in Table B5 in the Appendix.
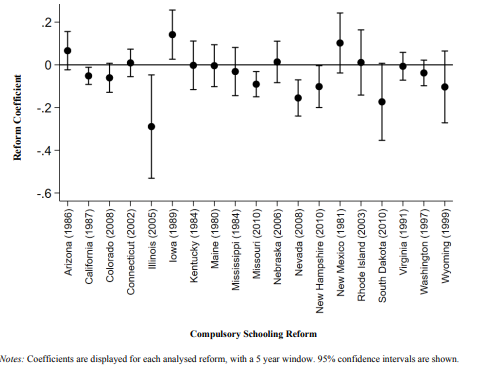
Gender Analysis
Finally, and most importantly for this research, the population is disaggregated and analysed by gender. These estimates are presented in Table 14
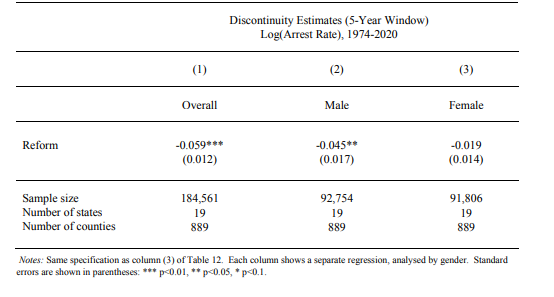
Column 2 reports significant negative results for males. The coefficient is similar to Bell, Costa and Machin (2022), though not as closely linked as for the fixed effects model. They find a reduction of 6%, compared to this paper’s finding of 4.5%. This suggests the omission of states with multiple reforms, and the incorporation of an additional 5 years of arrest data did have a slight change on regression discontinuity results.
The estimate for females is insignificant. Often, this is deemed to be due to the lower rates of female offences and is not examined further (see Machin, Marie and Vujic, 2011). However, in this case, the sample size is not hugely different, nor are the variances in the arrest data. The fact that a significant effect was found for females with the fixed effects model, but not when limiting analysis to a 5-year window either side of reforms, could suggest that the educational effects take longer to materialise for females than for males. To test this, I experiment with longer windows around each compulsory schooling law for the female data.
Table 15 presents the effects of the reforms on female crime for 7 and 10-year windows, extending the bandwidth from the previous analysis
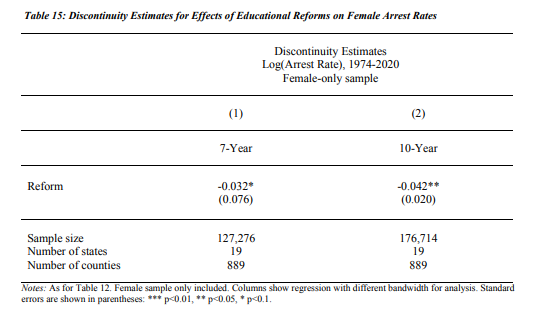
The results show estimates increasing in significance with the length of time period considered. Estimates for the 10-year window indicate a 4.2% reduction in crime from the reforms that is significant at the 5% level. Unlike in the fixed effects model, this is very similar to the estimate for male arrests.
Significant effects for female crime only being seen when examining longer windows around the discontinuity supports the idea that female education has a longer time lag, with immediate cohorts after the reform showing lesser crime reductions than those further away. The finding that female crime is affected more substantially than male is only revealed when the complete time period is considered (in the fixed effects model), implying that the full effects can take a long time to materialise.
This time-lag has also been found to exist for welfare reform impacts on female crime (Corman, Dave and Reichman, 2014). A time-lag between reforms and crime could be attributed to delayed compliance – initial compliance levels are unlikely to be perfect, with some students not obeying the new laws (Chib and Jacobi, 2016). It seems feasible for this compliance to increase with time, as gradually the new minimum drop-out age becomes the ‘norm’. However, the finding that there is a larger delay for females than for males, is harder to justify. At present, neither theory nor empirical evidence seems to provide sufficient information that could be used to explain the reasons behind it. Uncovering factors underlying this requires further empirical research.
In summary, the regression discontinuity design substantiates theory that educational reforms lead to significant reductions in crime. It offers added insight regarding the adjustment period between the enactment of reforms and the beneficial crime effects, revealing a longer time-lag for effects to show for female crime.
Overall, this paper succeeds in addressing the research question and demonstrating a causal link. However, it does have a significant limitation in its lack of ability to empirically distinguish the underlying channels linking education to lowered crime.
6. Conclusion.
The implications of this study support existing conclusions of a negative causal relationship between education and crime, with both fixed effects and regression discontinuity models indicating significant crime reducing effects of recent US education reforms for a sample encompassing both genders. This contributes to evidence that education has value as a method of crime prevention, and these resulting positive externalities mean the social returns to schooling exceed those directly affecting the economy through labour market returns and innovation. In terms of future policy, the evidence highlights that when evaluating the use of further compulsory schooling reforms, the social returns from crime must be taken into account as part of the benefits. The decomposition of impacts by the gender of the perpetrator gives additional insight into the nature of these effects. Our study mirrors the general findings from Cano-Urbina and Lochner (2019), who also find significant reductions for females, but use data pre-1980. Despite hypothesising that the effects are now likely to be more related to labour market channels than marital status, this paper is limited to the extent that it cannot provide empirical evidence to support this claim. Hence, further research should be conducted to test this. Further examination of these channels may also reveal reasons behind the finding that the effects take longer to materialise for females than for males, as existing research does not appear to provide evidence that could be used to explain this.
Another implication of this paper relates to gender-crime convergence, which acts as the motivating force behind this study, emphasising the importance of female inclusion in crime analysis. Although the study’s main aim is not to investigate the causes of this pattern, a by-product of the analysis is the elimination of the possibility that educational factors could be behind this. There is no evidence that male crime has been reduced more substantially by education reforms than female crime – in fact the reverse effect is found, which if anything, would widen the gender offence gap. The study of alternative social factors that could explain this phenomenon would be an interesting channel to direct future economic research, with all existing studies only being from a criminological perspective (Steffensmeier and Allan, 1996). This research would be useful both for theoretical purposes, but also for the practical element of developing polices that could help to reduce female crime at a more similar rate to males, decreasing overall crime by a greater margin.
7. References
Abadie, A., Athey, S., Imbens, G., and Wooldridge, J. (2017). When Should You Adjust Standard Errors for
Clustering? Working Paper 24003, National Bureau of Economic Research.
Anderson, M. (2014). In School and Out of Trouble? The Minimum Dropout Age and Juvenile Crime. The Review of
Economics and Statistics, 96(2): pp. 318–331.
Asante, G. and Bartha, A. (2022). The positive externality of education on crime: Insights from Sub-Saharan Africa.
Cogent Social Sciences, 8(1): pp. 1-24
Beatton, T., Kidd, M. P. and Machin, S. (2018). Gender crime convergence over twenty years: Evidence from
Australia. European Economic Review, 109: pp. 275–288.
Becker, G. S. (1968). Crime and Punishment: An Economic Approach. Journal of Political Economy, 76(2): pp.
169–217.
Becker, G. S. (1993). Nobel Lecture: The Economic Way of Looking at Behavior. Journal of Political Economy,
101(3), 385–409. http://www.jstor.org/stable/2138769
Becker, G. S. and Mulligan, C. B. (1997). The Endogenous Determination of Time Preference. The Quarterly
Journal of Economics, 112(3): pp. 729–758.
Bell, B., Costa, R. and Machin, S. (2016). Crime, compulsory schooling laws and education. Economics of Education
Review, 54: pp. 214–226.
Bell, B., Costa, R. and Machin, S. (2022). Why Does Education Reduce Crime? Journal of Political Economy,
130(3): pp. 732-765.
Bennett, P. (2018). The heterogeneous effects of education on crime: Evidence from Danish administrative twin data. Labour Economics, 52: pp. 160–177.
Bindler, A. and Hjalmarsson, R. (2017). Prisons, recidivism, and the age–crime profile. Economics Letters, 152: pp.
46–49.
Brunello, G., Fort, M. and Weber, G. (2009). Changes in Compulsory Schooling, Education and the Distribution of
Wages in Europe. The Economic Journal, 119(536): pp. 516–539.
Buonanno, P. and Leonida, L. (2009). Non-market effects of education on crime: Evidence from Italian regions.
Economics of Education Review, 28(1): pp. 11–17.
Buonanno, P. and Montolio, D. (2009). Juvenile crime in Spain. Applied Economics Letters, 16(5): pp. 495–500.
Cano-Urbina, J. and Lochner, L. (2019). The Effect of Education and School Quality on Female Crime. Journal of Human Capital, 13(2): pp. 188–235.
Card, D. (1999). The Causal Effect of Education on Earnings. In: Ashenfelter, O. C. and Card, D. (Eds). Handbook
of Labor Economics, Volume 3A, Chapter 30, pp. 1801-1863. Amsterdam: Elsevier.
Carpenter, C. and Dobkin, C. (2015). The Minimum Legal Drinking Age and Crime. The Review of Economics and
Statistics, 97(2): pp. 521–524.
Chen, J.-Y. J. and Giles, D. E. A. (2004). Gender convergence in crime: Evidence from Canadian adult offense
charge data. Journal of Criminal Justice, 32(6): pp. 593–606.
Chib, S. and Jacobi, L. (2016) Bayesian Fuzzy Regression Discontinuity Analysis and Returns to Compulsory
Schooling. Journal of Applied Economics, 31: pp. 1026-1047
Corman, H., Dave, D. and Reichman, N. (2014) Effects of welfare reform on women’s crime. International Review
of Law and Economics, 40: pp. 1-14
Cygan-Rehm, K. and Maeder, M. (2013). The effect of education on fertility: Evidence from a compulsory schooling reform. Labour Economics, 25: pp. 35–48.
di Tella, R. and Schargrodsky, E. (2004). Do Police Reduce Crime? Estimates Using the Allocation of Police Forces
after a Terrorist Attack. The American Economic Review, 94(1): pp. 115–133.
Dursun, B. and Cesur, R. (2016). Transforming lives: the impact of compulsory schooling on hope and happiness.
Journal of Population Economics, 29(3): pp. 911–956.
Eggers, A. C., Tuñón, G. and Dafoe, A. (2021). Placebo Tests for Causal Inference. Working Paper.
Ehrlich, I. (1975). On the Relation between Education and Crime. In F. T. Juster (Ed.), Education, Income, and
Human Behavior, Chapter 12, pp. 313-338. New York: McGraw-Hill Book Co.
Fajnzylber, P., Lederman, D. and Loayza, N. (2002). Inequality and Violent Crime. The Journal of Law and
Economics, 45(1): pp. 1–39.
Garofalo, J. (1981). The Fear of Crime: Causes and Consequences Criminology: Symposium on Victimization and
Victimology. Journal of Criminal Law and Criminology, 72(2): pp. 839–857.
Gelman, A. and Imbens, G. (2019). Why High-Order Polynomials Should Not Be Used in Regression Discontinuity
Designs. Journal of Business and Economic Statistics, 37(3): pp. 447–456.
Goldin, C. (2014). A Grand Gender Convergence: Its Last Chapter. American Economic Review, 104(4): pp. 1091–
1119.
Groot, W. and van den Brink, H. M. (2010). The effects of education on crime. Applied Economics, 42(3): pp. 279–
289.
Hamilton, B. E. and Ventura, S. J. (2006). Fertility and abortion rates in the United States, 1960–2002. International
Journal of Andrology, 29(1): pp. 34–45.
Hjalmarsson, R. (2008). Criminal justice involvement and high school completion. Journal of Urban Economics,
63(2): pp. 613–630.
Hjalmarsson, R., Holmlund, H. and Lindquist, M. J. (2015). The Effect of Education on Criminal Convictions and
Incarceration: Causal Evidence from Micro‐data. The Economic Journal, 125(587): pp. 1290–1326.
Hungerman, D. M. (2014). The effect of education on religion: Evidence from compulsory schooling laws. Journal
of Economic Behavior and Organization, 104: pp. 52–63.
Ionescu, A. (2012). How does education affect labour market outcomes. Review of Applied Socio-Economic
Research, 4(2): pp. 130–144.
Jacob, B. A. and Lefgren, L. (2003). Are Idle Hands the Devil’s Workshop? Incapacitation, Concentration, and
Juvenile Crime. American Economic Review, 93(5): pp. 1560–1577.
Kemptner, D., Jürges, H. and Reinhold, S. (2011). Changes in compulsory schooling and the causal effect of
education on health: Evidence from Germany. Journal of Health Economics, 30(2): pp. 340–354.
Kling, J. (1999). The Effect of Prison Sentence Length on the Subsequent Employment and Earnings of Criminal
Defendants. Discussion Paper 208, Princeton University.
Kravdal, Ø. and Rindfuss, R. R. (2008). Changing Relationships between Education and Fertility: A Study of
Women and Men Born 1940 to 1964. American Sociological Review, 73(5): pp. 854–873.
Lin, M.-J. (2009). More police, less crime: Evidence from US state data. International Review of Law and
Economics, 29(2): pp. 73–80.
8. Appendices
Appendix A: Additional Data Description
Application of coverage ratios
Arrest rates are calculated by dividing the UCR data by the SEER age-sex-county population estimates. Due to the aforementioned inconsistent reporting of enforcement agencies, it would be inaccurate to use these population statistics without prior adjusting – if only a small proportion of agencies within a county actually report their arrests, using this simple method would grossly underestimate the true arrest rates.
To tackle this issue, county population statistics must be adjusted according to the proportion of the county represented by included agencies. This relies on the assumption that the missing population (covered by agencies that do not consistently report) has approximately the same demographic characteristics as the overall county population.
Collating the UCR population count for each included agency by county-year, and dividing this by the official SEER population data for the county as a whole enables the identification of a countyyear covering ratio. This averages 76.2% for the dataset. These county-year coverage ratios are then applied to the SEER population estimates. The resulting age-sex-county population statistics are used as weights in all regressions, and are now valid to be used in the calculation of arrest rates.
Control Variables
Police Numbers
The FBI LEOKA database provides the total number of sworn officers yearly for each law enforcement agency. These figures can be aggregated at a county level to measure overall police presence. The inclusion of this variable is motivated by the vast literature showing significant interactions between police and crime rates, including Weisburst (2019) and Lin (2009).
Racial and sex breakdown
The same method used to obtain age-sex population cells for arrest rate calculation is used to estimate the racial and gender breakdown of each county in each year. SEER provide yearly county population approximations by race (grouped into 3 categories of black, white and non-white/nonblack) and gender. I applied the county coverage ratio to these estimates to scale for extent of reporting, and inputted these figures as a percentage of the UCR population.
School Attendance
School attendance statistics for those aged 16-18 can be retrieved from The National Bureau of Economic Research archive, containing data from the Current Population Survey from 1976 to 2020. “Attendance” refers to the proportion of subjects enrolled full-time in high school, conditional on not attending superior education. This is used only for confirmation of instrument relevance – in all further regressions, compulsory schooling laws are used to instrument for education rather than using attendance figures
Appendix B: Supplementary Tables.
Table B1: Summary of core empirics
Author(s)/Year | Title | Country | Time period studied | Data | Methodology |
---|---|---|---|---|---|
Ehrlich (1975) | In relation to education and crime | US | 1960 | Individual-level cross-sectional data from US Census and state level data from FBI UCR | OLS regression using state and individual controls. |
Lochner and Moretti (2004) | The Effect of Education on Crime: Evidence from Prison Inmates, Arrests, and Self-Reports | US | 1960-1980 | Individual-level US Census Data and NLSY, state-level panel data from FBI UCR | IV estimation using US State Compulsory Schooling Reforms |
Machin, Marie and Vujíc (2011) | The Crime Reducing Effect of Education | UK | 1963-1996 | Panel data (anonymous sample) from UK Offenders Index Database | IV estimation and regression discontinuity using UK 1972 Schooling Reform |
Hjalmarsson, Holmlund and Lindquist (2015) | The Effect of Education on Criminal Convictions and Incarceration: Causal Evidence from Micro-Data | Sweden | 1973-2007 | Micro-data using Sweden’s Multigenerational Register and arrests from Sweden’s National Council for Crime Prevention | IV estimation using Swedish Compulsory Schooling Law changes |
Cano-Urbina and Lochner (2019) | The Effect of Education and School Quality on Female Crime | US | 1960-1980 | Individual-level data from US Census and state level data from FBI UCR | IV estimation using US State Compulsory Schooling Reforms |
Bell, Costa and Machin (2022) | Why does Education Reduce Crime? | US | 1974-2015 | Police agency level data from FBI UCR (aggregated at county level) | OLS with Fixed Effects and Regression discontinuity using US State Compulsory Schooling Reforms |
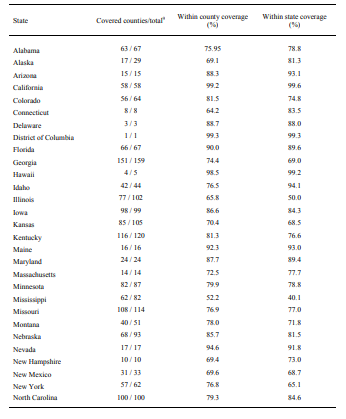
In some states, divisions are not officially called ‘counties’. For example, Alaska is divided administratively into boroughs.
However, these are still assigned FIPS county codes, so can be included and are named counties throughout for simplicity.